Google and Meta have made notable updates to their AI models, showcasing advancements that could reshape how businesses and developers leverage artificial intelligence. Google has rolled out upgrades to its Gemini model lineup, offering improvements in both speed and accuracy, while Meta has unveiled its updated Llama 3.2 models, enhancing capabilities in text and visual tasks. These developments arrive amid Google’s launch of AlphaChip, a tool designed to revolutionize the design of AI-accelerating chips. Here’s what these updates mean for AI.
What’s Happening & Why This Matters
Google’s Gemini Updates: Faster, Smarter, and Cheaper
Google has introduced two new models, Gemini-1.5-Pro-002 and Gemini-1.5-Flash-002, which build on previous releases with a focus on improving quality in areas like mathematics, long-context handling, and vision tasks. Notably, the updated models show a 7% improvement on the MMLU-Pro benchmark and a 20% increase in performance for math-related tasks. While benchmarks can be unreliable, these improvements hint at better usability for developers and businesses relying on AI for complex problem-solving.
The cost to use Google’s Gemini 1.5 Pro has also dropped significantly—64% lower for input tokens and 52% lower for output tokens on tasks under 128,000 tokens. This reduction in cost, combined with increased speed (double the output speed and three times lower latency), positions Gemini as a competitive option for developers looking for cost-effective AI solutions. Developers can now send up to 2,000 requests per minute with Gemini 1.5 Flash, making it faster and more efficient for large-scale applications.
Meta’s Llama 3.2: Enhanced for Vision and Text
Meta has responded with the release of Llama 3.2, which introduces vision-capable models alongside smaller text-only versions. These new models, ranging from 1 billion to 90 billion parameters, cater to both edge devices like smartphones and larger applications requiring heavy computational power. Meta claims the vision models can compete with leading image-recognition systems, while the text-based models outperform competitors of similar size on various tasks.
One notable feature is the ability to use Llama 3.2 on mobile devices, as demonstrated by AI researcher Ethan Mollick, who ran the model on his iPhone using an app called PocketPal. Meta has also released a “Llama Stack”, designed to simplify AI development and deployment across multiple platforms.
AlphaChip: Google’s AI-Designed Chip Layouts
In addition to its AI model updates, Google has made waves with the introduction of AlphaChip, a tool designed to optimize the layout of AI chips. Initially launched as a research project in 2020, AlphaChip uses reinforcement learning to design high-quality chip layouts in a fraction of the time it takes human engineers. Google reports that AlphaChip has been used to design three generations of Tensor Processing Units (TPUs) and claims it can achieve in hours what typically takes engineers weeks or months.
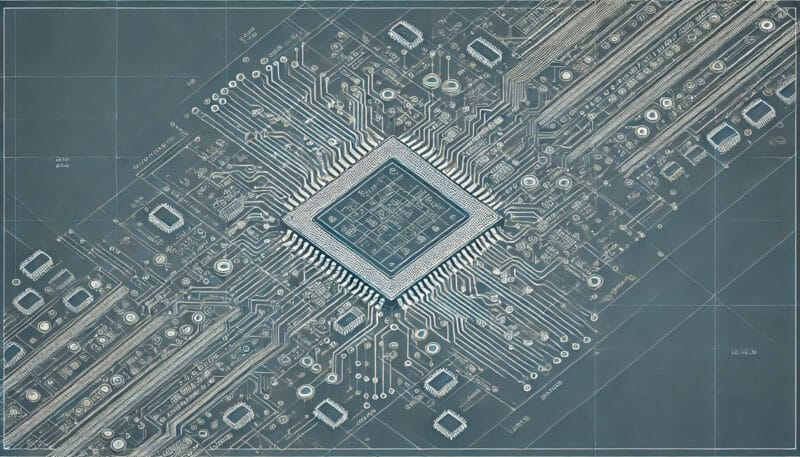
Companies like MediaTek have already started adopting AlphaChip to enhance their chip designs, signaling that the tool could become a staple in the semiconductor industry. The release of AlphaChip’s pre-trained checkpoint on GitHub also opens the door for wider use and further research.
TF Summary: What’s Next?
The updates from Google and Meta showcase the rapid pace at which AI technology is evolving. Google’s Gemini models are now more affordable and efficient, making them an attractive option for developers. Meanwhile, Meta’s Llama 3.2 models offer versatility across text and visual tasks, with a particular emphasis on mobile and edge applications. Additionally, Google’s AlphaChip promises to streamline AI hardware development, potentially accelerating innovation across the tech industry. As AI models and tools improve, businesses and developers stand to benefit from more accessible and powerful AI solutions.