Speed and accuracy are key when developing and refining AI models. Enter Databricks, a platform designed to help data scientists and engineers build, train, and deploy AI models more efficiently. Whether you’re working with massive datasets or fine-tuning existing models, Databricks offers tools that speed up the process and improve outcomes.
What’s Happening & Why This Matters
Databricks is quickly becoming a favorite among data engineers and scientists for its ability to simplify and accelerate the process of building AI models. One of its standout features is its unified analytics platform, which allows teams to collaborate in real-time. This feature is handy for working with large datasets that are often too complex to manage with traditional methods.
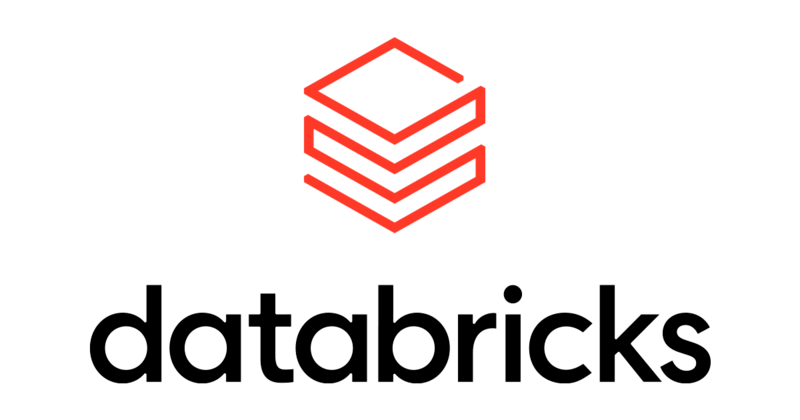
Apache Spark, the engine behind Databricks, is designed to handle large-scale data processing. This is ideal when working with data that comes from multiple sources, such as cloud storage or IoT devices. Databricks integrates this engine seamlessly into its platform, making it easy to process and analyze data in real-time.
In addition to its powerful data processing capabilities, Databricks supports machine learning and deep learning workflows. It offers a variety of pre-built tools and libraries that help automate tasks like feature engineering, model training, and deployment. For example, Databricks supports popular frameworks like TensorFlow, PyTorch, and scikit-learn, so you can easily integrate them into your workflow.
The platform also streamlines model management. It helps you monitor model performance and retrain models as new data becomes available. This ability to rapidly adapt your models to changing data conditions is essential for industries like finance, healthcare, and e-commerce, where predictive accuracy is crucial.
One of the reasons Databricks is so efficient is its collaborative environment. Data scientists and engineers can work together within the platform, reducing the need for lengthy back-and-forth between teams. This cooperative aspect, combined with its real-time data processing, ensures that teams can deploy better models faster.
Furthermore, Databricks is cloud-native, meaning it’s built for cloud-based environments like AWS, Azure, and Google Cloud. This enables teams to scale their operations without worrying about infrastructure limitations. With the ability to quickly spin up and shut down resources, Databricks optimizes cost and performance.
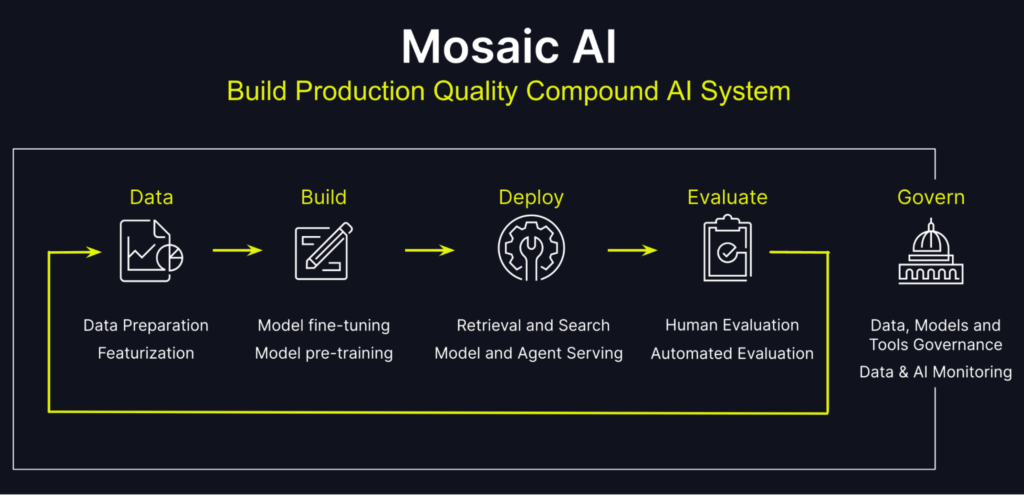
TF Summary: What’s Next
The need for faster, more efficient AI tools is increasing as capabilities and utilization balloon. Databricks provides a powerful solution for teams looking to accelerate their model-building processes. By offering a unified platform that combines data engineering, machine learning, and real-time collaboration, Databricks is making it easier for teams to build AI models that deliver results. The future of AI development is all about speed and collaboration, and Databricks is helping drive that change.
— Text-to-Speech (TTS) provided by gspeech